Thinking like AI: A new approach to AI UX design
What if AI had a resume? Our latest UX research exercise revealed fresh insights into AI’s role, challenges, and future in UX design.
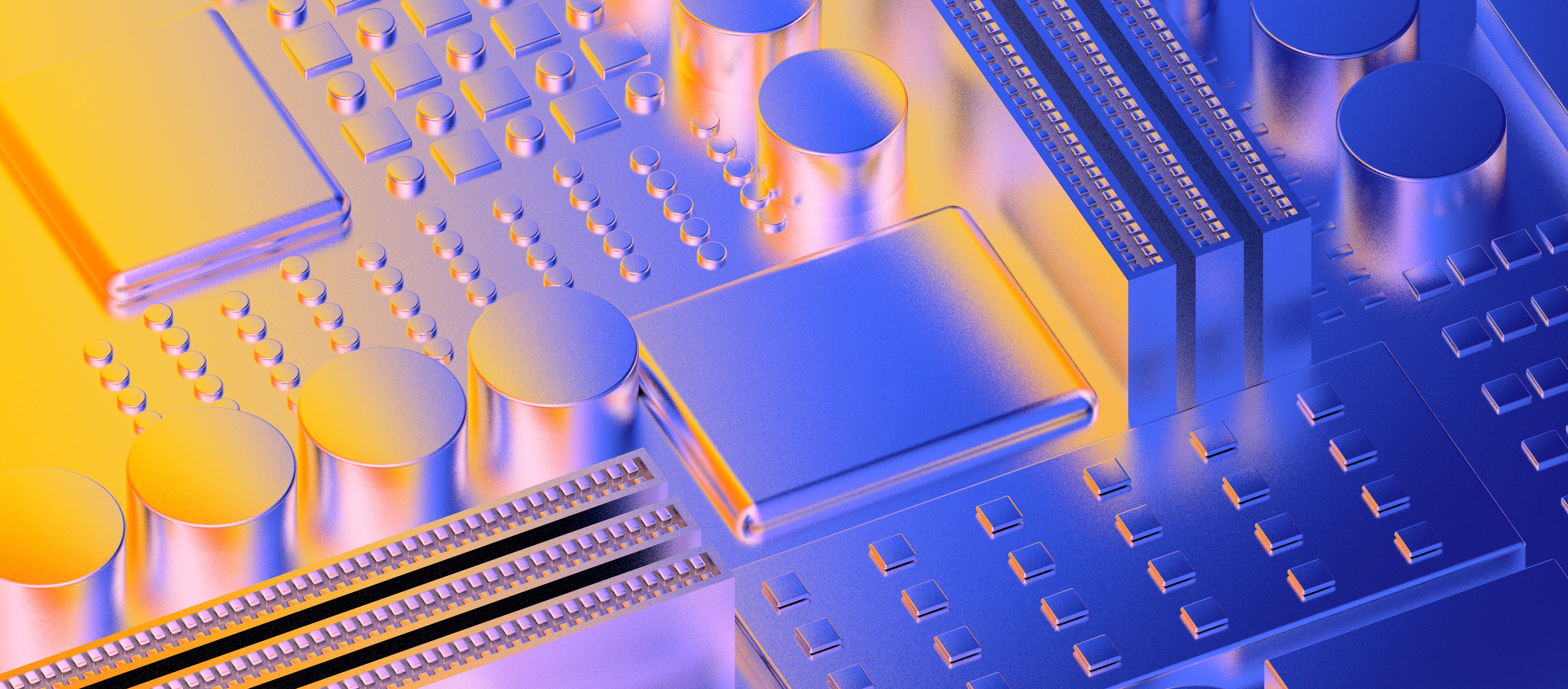
As product makers develop more and more AI features, it’s important to not only think about our human users’ requirements and preferences, but also those of the AI supporting them. To do this, I recently trialled a fun and collaborative method with my product team at Microsoft, a multidisciplinary group of Designers, PMs, and Engineers working on AI features in Microsoft Dynamics 365. I’m excited to share my process here for anyone else who might like to give it a try.
Context
Think about reading this article on Microsoft Design right now.
• Why are you ‘hiring’ the site?
• What ‘job’ is it helping you with and what is your goal?
• What tasks do you perform to achieve that goal?
• What pain points and challenges do you encounter?
For me, I might say that I hire different blogs to help me with the job of learning because I want to gain an edge in my career. To do this, I frequently check for new articles, add appealing ones to my reading list, read them, and hope I don’t discover halfway through that an article is less helpful than I’d hoped.
Here, I’ve effectively described a job-to-be-done (JTBD), its sub-tasks, and an associated pain point. At Microsoft, this JTBD framework is typically how we think about our business users. For my team, those are Enterprise Resource Planning (ERP) customers— everyone from accountants to supply chain managers—who ‘hire’ our Dynamics apps to help them do their work. Over the years, we’ve developed interactive persona cards for these various users, allowing team members to get a quick overview or drill into each of their JTBD to understand the corresponding tasks, success metrics, pain points, and more. Using this framework helps us to think holistically about our users and their goals, so we can design features and experiences that allow them to navigate our products as effectively and efficiently as possible.
Of course, like many teams in tech right now, we’re deeply focused on AI features. To find a good fit for AI features, we need to have empathy for our users and identify jobs and sub-tasks where AI might help them, rather than forcing it into the product. However, I think we also need to have empathy for the AI itself. If we want our AI features and autonomous agents to navigate our products as effectively and efficiently as our human users do (leveraging the right data, surfacing the right insights, etc.), perhaps we should also think about AI’s jobs-to-be-done, motivations, pain points and preferences.
If you’re on board, you might be thinking, “how?” JTBD research typically involves interviewing users, mapping out common themes into jobs and tasks, associating different pain points or challenges, and then maybe even validating or refining the results with a large-scale survey. Sure, we could try interviewing Copilot, ChatGPT or other LLMs, but their view is rather limited to what’s already been done—not where we’re trying to go in the coming years.
Data Collection
To overcome this issue, I held a workshop with fifteen colleagues across the ERP suite of products, with representatives from Design, PM, and Engineering. I asked participants to imagine themselves as the AI for our ERP product suite, three years in the future. I played some light background techno music and team members shared gifs of robots in the chat to help get into the mindset. Once ready, I provided the scenario:
“You are the AI that supported Microsoft’s ERP users for the past three years. Now, you’re sick of it and you want a new job. You decide to apply to be the AI for ERP users at another company. Let’s start by writing the experience and responsibilities section of your CV/resume.”
Workshop participants were given 10 minutes and their own individual templates to complete the task. As they worked, I picked out interesting sticky notes and highlighted them in the chat, encouraging people to stay motivated and engaged. Examples included “I automated repetitive tasks” and “I helped users to write better reports.”
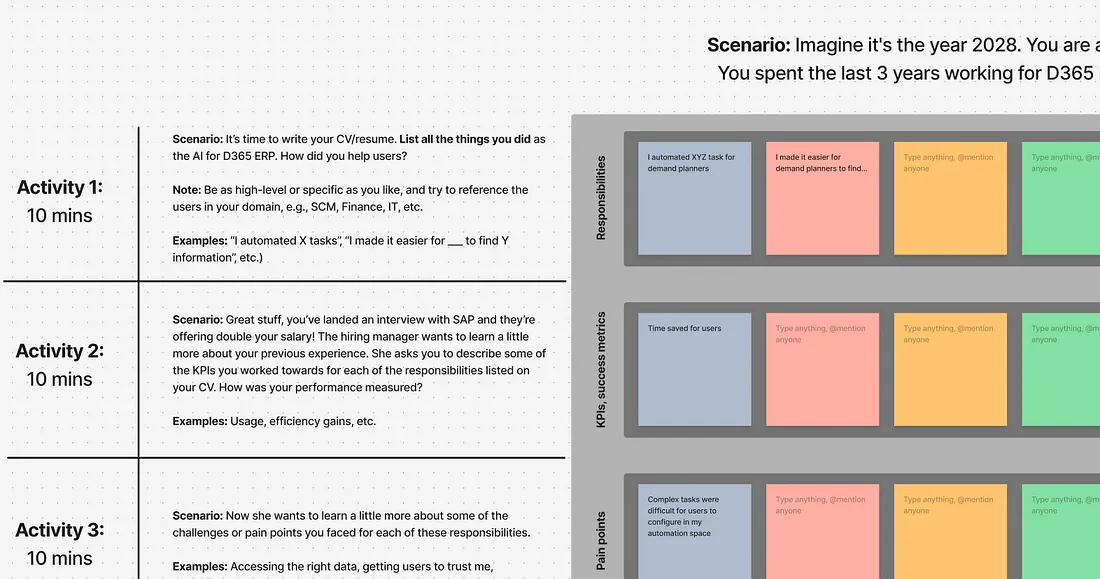
For the remaining activities, I told participants we had landed an interview with one of our competitors. The hiring manager first wanted to learn more about the success metrics for each of the responsibilities they had previously listed on their CV, giving examples like “time saved” and “adoption rate.” The hiring manager then wanted to know more about the pain points or challenges they (as an AI) had experienced in their previous role, like “gaining users’ trust” and “ambiguous prompts.” They also wanted to know their requirements if they were to become the AI for this company’s users, like “clear, structured datasets” and “AI onboarding material for users.” Finally, as with many interviews, the hiring manager wanted to know where they saw themselves in ten years, forcing participants to think far into the future about how AI might support our users.
Participants really enjoyed the workshop, and it encouraged the various disciplines to think in a different way than they otherwise might in their day-to-day. For example, engineers were forced to really focus on the users and the different ways AI might benefit them, while designers had to think technically about the challenges AI might encounter with data quality and app architecture. Encouraging everyone to think from the AI’s point of view gave us a fresh perspective on the opportunities and challenges ahead.
Analysis
The workshop yielded a lot of diverse content (400+ sticky notes) for me to organise, clean, analyse, and ultimately translate into an AI persona card. Fortunately, some careful planning of the workshop material made this a breeze and allowed me to quickly generate two sets of results to compare.
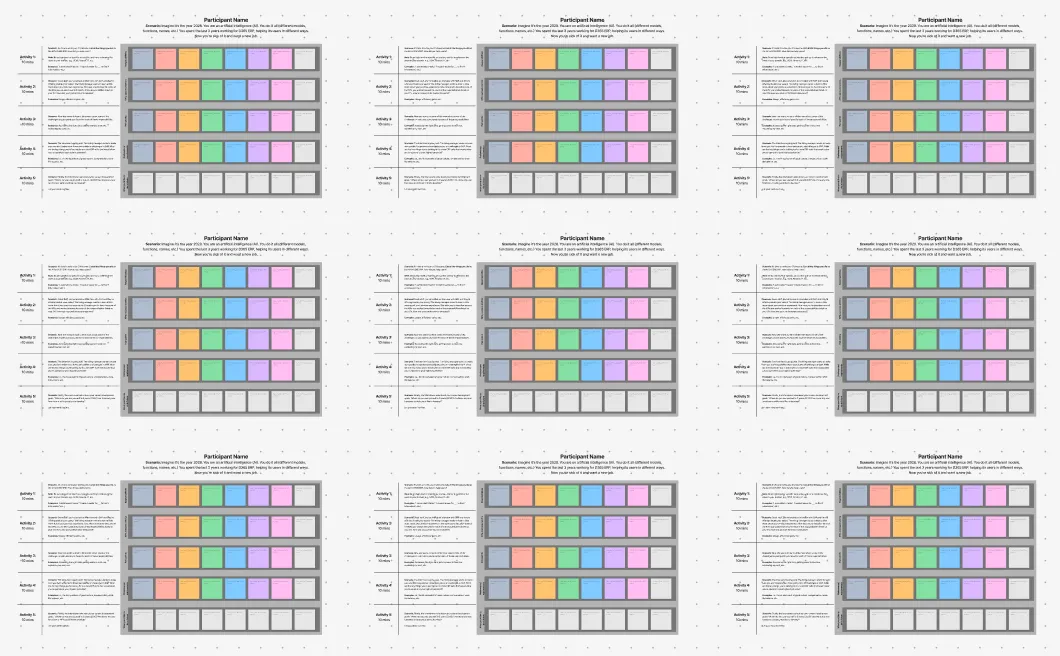
First, because the grids had encouraged participants to keep their content aligned by columns and colour, I could group whole sets of sticky notes together, rather than just individual ones. For example, if one participant described ‘automating tasks’ as a responsibility and another described ‘doing repetitive work autonomously,’ I could group these together under the JTBD of ‘Automation,’ but could also group the associated tasks, success metrics, pain points, and challenges under them.
Second, the grid format meant that I could also easily copy/paste the content into an Excel template and ask Copilot to extract the findings for me.
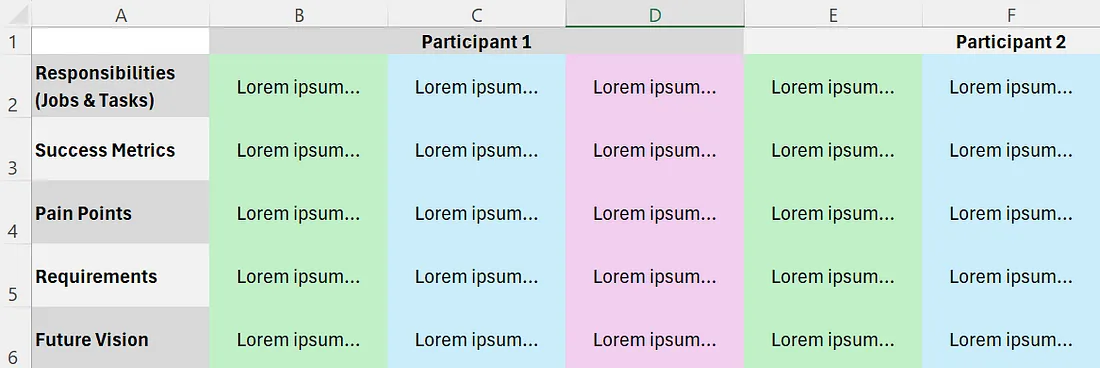
I simply used a prompt like:
“I’m a UX Researcher aiming to define the JTBD, tasks, success metrics, pain points, requirements and future goals for AI in ERP. I conducted a workshop with fifteen team members where they imagined themselves as this AI three years into the future, reflecting back on their work as they wrote their resume and interviewed for a job. Here is the raw data from the workshop, organised by columns (i.e., the success metrics, pain points, and requirements defined in B3, B4 and B5 all relate to the responsibility described in B2). I want you to analyse the data and present your findings in the following format:
- JTBD 1
- Tasks
- Success metrics
- Pain points
- Requirements
- Repeat for other JTBD
- Summary of future visions across JTBD”
Once Copilot had done its analysis, I could compare its output to my own findings to see if we had arrived at similar conclusions. Before running the workshop, I had expected the JTBD to tie directly to the different types of AI features the team had been exploring, like autonomous agents and chat, but found that this wasn’t really the case after the workshop. Fortunately, Copilot also arrived at a similar number of JTBD that worked horizontally across these different types of AI features, providing reassurance in the analysis. In fact, it did an even better job of wording the JTBD and related information at a consistent level, so I adopted many of its recommendations when translating the results into an AI persona card.
Conclusion
While this project was only recently carried out, many product teams are excited about the AI persona card and plan to use it to support their AI developments for the upcoming year. Rather than focusing only on the user’s JTBD when developing a new feature, they’ll also refer to AI’s relevant JTBD and ensure they are considering its tasks, success metrics, pain points and requirements, so they can provide an experience that is optimum for both the human user and the AI to navigate.
Artwork by Karan Singh.
Read more
To stay in the know with Microsoft Design, follow us on Twitter and Instagram, or join our Windows or Office Insider program. And if you are interested in working with us at Microsoft, head over to aka.ms/DesignCareers.
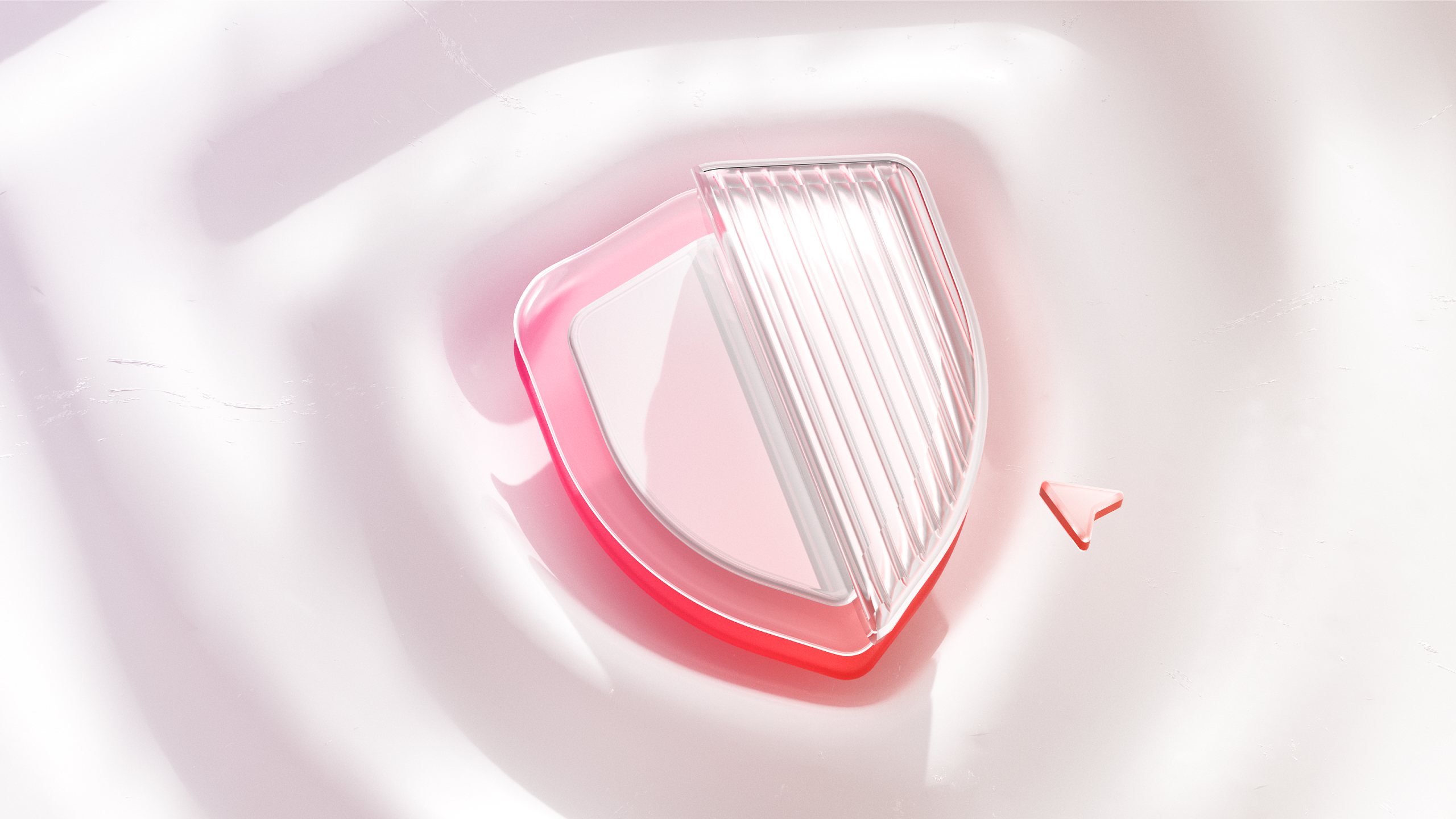
Secure by design: a UX toolkit
Human error causes a majority of security breaches. Our UX toolkit can help you build secure and empowered user experiences.
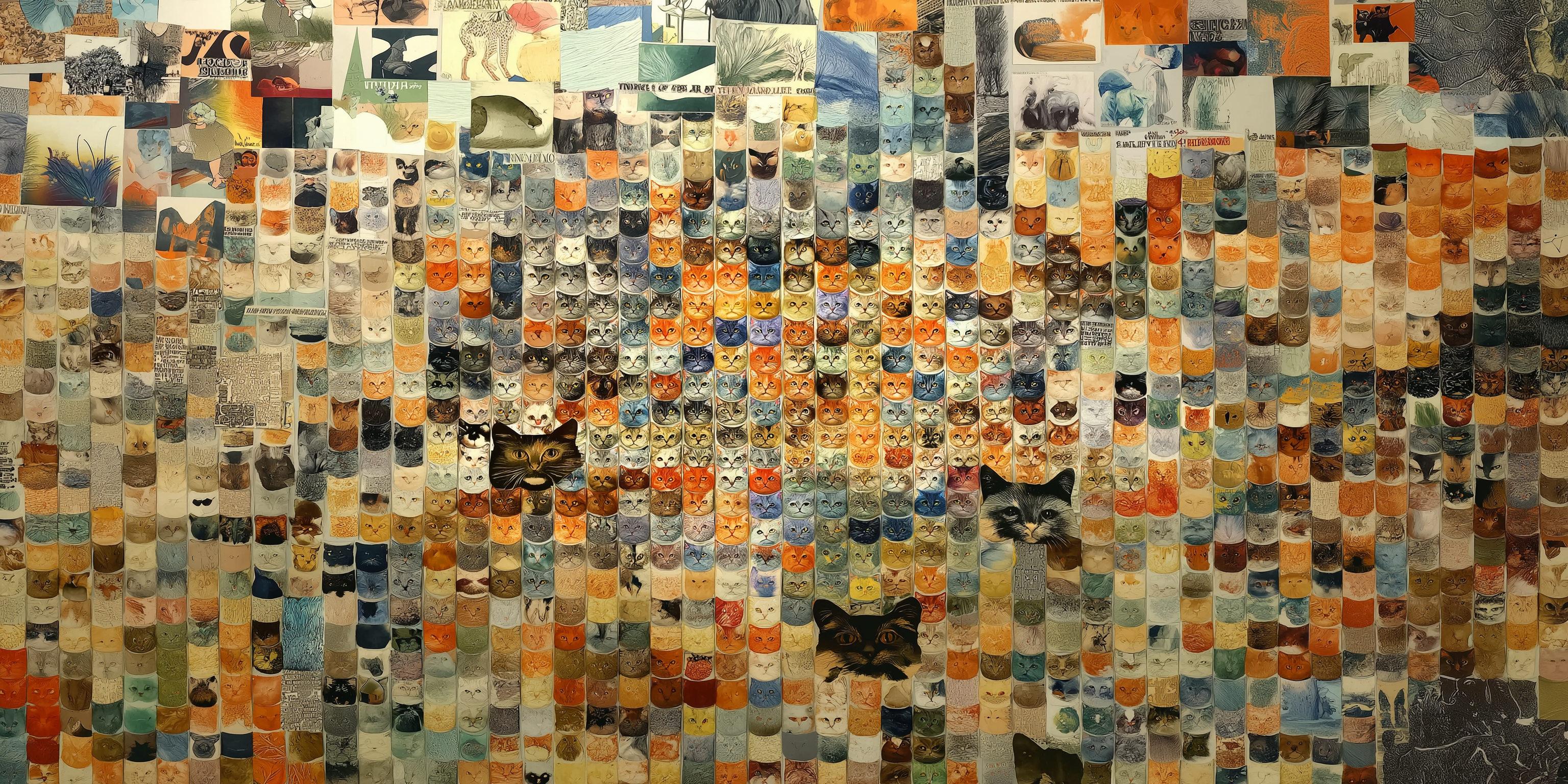
Lucid dreaming together with AI
A trip to Sintra that pushed the boundaries of my creativity